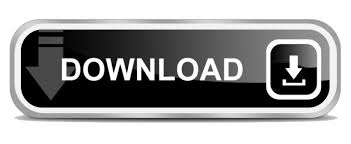
With that said, systems like AlphaGo are genuinely exciting. DeepMind used machine learning and neural networks to give its AlphaGo the ability to learn and play at a world-class level. At the moment, there are many applications and fields in AI are applied these technologies, such as in Natural Language Processing, Image and Speech Recognition…, etc. That made the Google’s Go win is a such a big deal in AI history.ĪlphaGo’s victories against the Korean legendary Go player in 2015 marked a major milestone in AI research. Since DeepMind’s AlphaGo has ability to learn based on machine learning technologies, it is a lot more human-like and, of course, artificially intelligent than IBM’s Deep Blue, which beat chess master Kasparov by using brute forte computing power to search for the best moves. I will have another post on deep learning and neural networks soon. AlphaGo’s intelligence is based on its ability to learn from millions of Go positions and moves from human-played games. This is the same technology that Facebook uses to recognize faces in images that have been processed down to pixel level. What is behind AlphaGo? How did DeepMind’s AlphaGo do it?ĪlphaGo uses deep leaning and neural networks to essentially teach itself to play. In 2015, AlphaGo, the Go-playing system defeated Lee Sedol, one of the strongest Go players in the world. DeepMind’s AlphaGo takes AI to a new level Therefore, its state space complexity makes Go hard for computer to play and becomes a big challenge for AI researchers. Traditional AI methods, which construct a search tree over all possible positions, don’t have a chance in GoĢ. The possible positions of Go is more than a googol (10^100) times larger than in chess. The reason is Go is a game of profound complexity. Why the search strategy cannot be applied for Go? It seemed as though a strong intuitive sense of board position was essential to success. But Go programs still fell far short of human players in ability. This began to change in 2006, with the introduction of so-called Monte Carlo tree search algorithms, which tried a new approach to evaluation based on a clever way of randomly simulating games. Though, after decades of attempts to do this using conventional approaches, there was still no obvious way to apply the search strategy that was so successful for chess, and Go programs remained disappointing. You might think it’s just a question of working hard and coming up with a good way of evaluating board positions. It just simply used its advantage in high performance computing to calculate millions calculations in a second. That’s it.īut, can we apply the same technique for Go? Until now, the answer is NO. So, here you could think that Deep Blue can not be called “smart”. Second, they used an IBM’s supercomputer to find the best possible positions, picking out the move that would force the best possible final board position. First, they built a function that incorporated lots of detailed chess knowledge to evaluate any given board position. IBM’s programmers use the two main ideas above for their Deep Blue program. To the the sub-problem (ii), there will be various answers which depends on the game and the cleverness of solver. Normally, programmers use minimax search with alpha-beta pruning or other heuristics algorithms for searching. In general, we have to deal with two major sub-problems, that’s (i) searching in the state space and (ii) finding the best evaluation function for the game.įor the games like Chess and Caro, the sub-problem (i) can be solved by using a wise optimization strategy and a fast enough computer. Computer Science did not undergo a revolution.Īn overview of Artificial Intelligence’s techniques for GamesĬonsidering the games of two opposing opponents like Chess, Caro or Go…, the answer to these problems can be referred to the state space search or combinatorial optimization problem. Though, Deep Blue’s technology turned out to be useful for chess only and not much else. At that time, the victory was widely described as milestone in AI. It was the first time that the world witnessed the historic event of a computer being worthy contender to one of the world’s sharpest mind. In 1997, the Deep Blue system of IBM defeated the world chess champion, Gary Kasparov. Deep Blue, An Artificial Intelligence Milestone What are the difference between the two systems and its meaning to the development of AI? This article will provide you with some fundamental information to answer this question.ġ. IBM’s Deep Blue (Deep Blue) and Google DeepMind’s AlphaGo (AlphaGo) are the two famous computer programs in the history of Artificial Intelligence (AI).
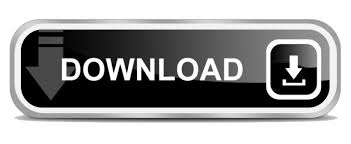